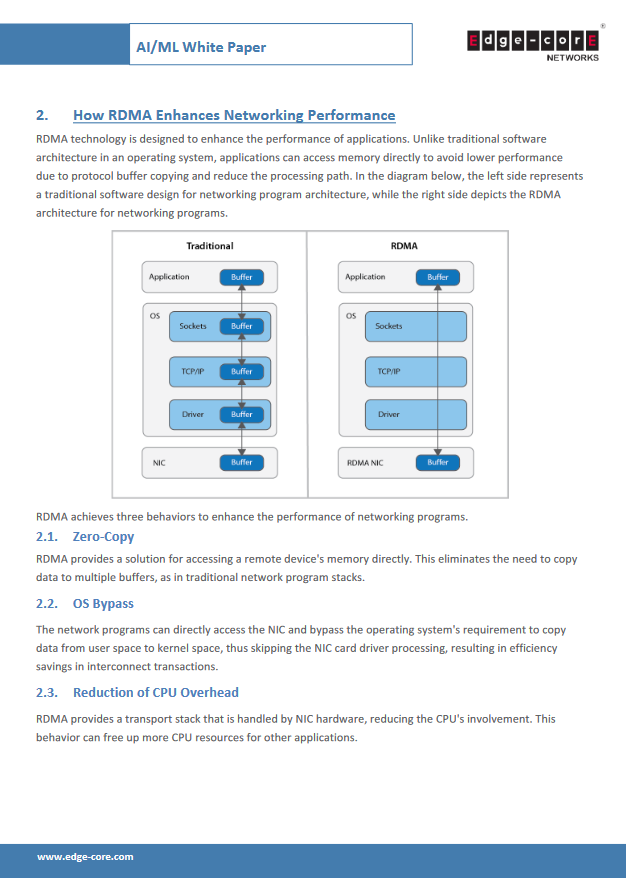
Overview
In recent years, the demand for Artificial Intelligence and Machine Learning (AI/ML) capabilities in data centers has been increasing. Most applications now leverage deep machine learning with deployment on distributed neural networks. This approach ensures that resources remain unblocked and calculate in parallel, allowing for seamless scaling to meet increasing services demands. In such high-speed network environments, DCQCN(Data Center Quantized Congestion Notification) stands as a pivotal congestion control algorithm in RoCEv2 networks, effectively combining ECN (Explicit Congestion Notification) and PFC (Priority Flow Control) to facilitate end-to-end lossless Ethernet.
There is one main difference between AI/ML networking and cloud networking; there are more elephant flow cases in AI/ML. In other words, higher speeds are needed to tolerate the data flow peak and address the growing distributed computing traffic. Regarding these challenges, a way needs to be found to tune the lossless and low-latency network in a higher-speed environment. This challenge can be approached from two perspectives: a compute node view and a communication network view.
- Download
- Download 220
- File Size 381 KB